Table of Contents
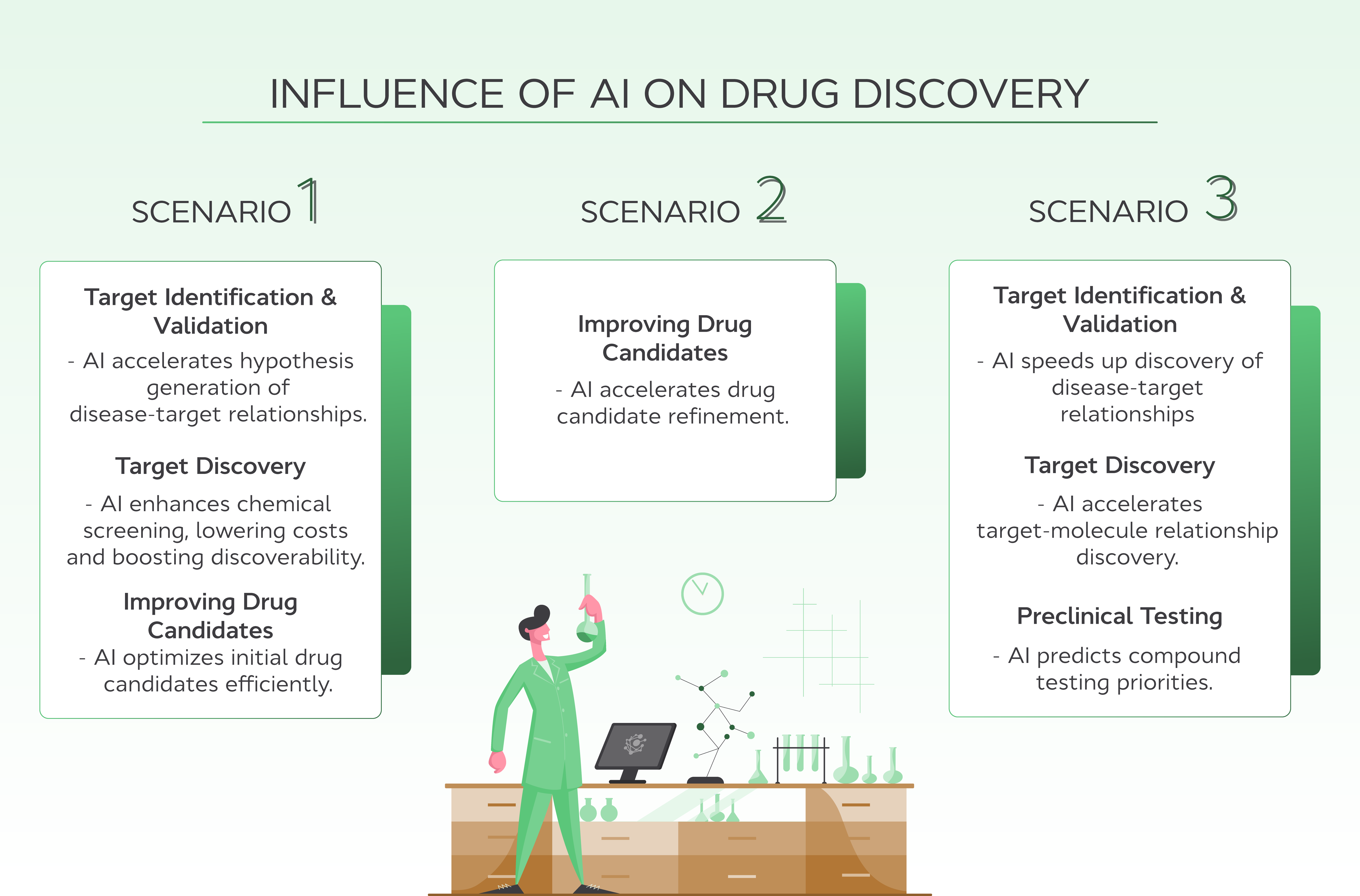
How AI-Driven Drug Discovery Could Transform the Pharma Industry
AI can accelerate drug discovery for pharmaceutical companies. But, AI technologies need to be trained on the right data for the process to work effectively. This article explains how OvalEdge can help pharmaceutical pioneers identify and utilize that data quickly and securely.
Fast-Track Failures
Researchers in the pharmaceutical industry have long sought to fast-track drug discovery. However, instead of achieving this ambition, this critical process has become slower, more expensive, and increasingly difficult.
Today, it usually takes between 12 and 15 years for researchers to turn the findings of a drug discovery program into a regulated drug available to patients. In fact, around nine out of ten drugs that reach the clinical trial phase don't receive regulatory approval.
And, in terms of financial impact, the numbers are huge. On average, it costs around USD $2.5 billion to see a drug to market, taking into account the combined expense of successful and unsuccessful trials.
AI Can Accelerate Drug Discovery
One particularly impactful report, co-authored by BCG and the research funding organization Wellcome, concludes that AI could generate “time and cost savings of at least 25–50%” up to the preclinical stage in drug discovery.
According to the BCG/Wellcome report, and in line with the thinking of many industry experts, AI has the potential to impact traditional drug discovery in multiple ways.
The following scenarios illustrate the profound influence AI can have on drug discovery:
Scenario one: New molecule for difficult or poorly understood molecular target
-
Target identification and validation: AI uses data mining and protein modeling to understand how targets relate to diseases quickly, speeding up hypothesis generation.
-
Target discovery: AI enables the screening of large chemical libraries at a lower cost than traditional methods, increasing the chances of finding targets and promising compounds for difficult use cases.
-
Improving drug candidates: AI reduces the number of compounds and experiments required to optimize initial drug candidates. However, it does rely on existing clinical data for new molecules.
Scenario two: Molecule from existing chemical series for well-understood molecular target
-
Improving drug candidates: For well-understood targets with ample existing data, AI cuts down on the number of cycles required to refine initial drug candidates.
Scenario three: Repurposing an existing drug for another molecular target
-
Target identification and validation: AI accelerates the discovery of disease-target relationships using data mining and patient data.
-
Target discovery: AI speeds up the discovery of new target-molecule relationships, though screening licensed libraries can add costs.
-
Preclinical testing: AI predicts toxicity and pharmacokinetics/pharmacodynamics, prioritizing compounds for testing and leveraging existing data for repurposed drugs.
However, AI cannot perform without correct data
Researchers use AI to accelerate drug discovery. However, that will only happen if the data used to inform these AI algorithms is correct. Ref:
Lack of high-quality data sets, access to mature tools, and relevant AI and drug discovery capabilities constrains the value being delivered from AI today. Source: BCG/Wellcome report
In fact, the availability of sufficient/high-quality data was the most common barrier to increasing the usage of AI tools in drug discovery, as per the BCG/Wellcome report. A massive 64% of the respondents cited this as a significant roadblock.
Let's explore how this issue of substandard data manifests across each of the three scenarios we highlighted earlier in this article.
Related Post: 4 Steps to AI-Ready Data
Scenario one: New molecule for difficult or poorly understood molecular target
-
Target identification and validation: Data limitations, such as incomplete or inconsistent datasets, can hinder AI's ability to identify and validate new disease-target relationships accurately. Without robust data, AI may struggle to generate reliable hypotheses.
-
Target discovery: Limited data quality or availability can restrict the effectiveness of AI when screening large chemical libraries. Inadequate data can lead to missed opportunities or inaccurate predictions in identifying promising targets and compounds. Open-source datasets often lack depth, dimensionality, and scale for the application of AI. For example, missing metadata on cell culture conditions.
-
Improving drug candidates: AI heavily relies on existing clinical data to optimize initial drug candidates. Insufficient or biased clinical data can hinder AI's ability to refine drug candidates efficiently, potentially delaying drug development progress.
Scenario two: Molecule from existing chemical series for well-understood molecular target
-
Improving drug candidates: While existing targets may have ample data, biases or gaps in the data can hinder AI's ability to optimize drug candidates. Inconsistent or incomplete data can lead to suboptimal decisions in refining drug designs.
Scenario three: Repurposing of an existing drug for another molecular target
-
Target identification and validation: Data issues such as limited availability of comprehensive datasets or biased patient data can make it difficult for AI technologies to discover new disease-target relationships. Inaccurate or incomplete data may lead to incorrect conclusions about potential drug repurposing opportunities.
Many databases contain inconsistencies in data structure, metadata, and normalization. This makes it challenging to apply AI techniques and amalgamate data to drive better target-disease hypotheses. -
Target discovery: Screening licensed libraries requires high-quality and diverse datasets. If the data used for training AI models is inadequate or unrepresentative, AI may struggle to identify suitable targets for repurposing existing drugs.
-
Preclinical testing: AI's ability to predict toxicity and pharmacokinetics / pharmacodynamics relies heavily on robust and comprehensive data. Incomplete or biased data can compromise predictions, leading to inaccurate drug safety and efficacy assessments during preclinical testing. Proprietary datasets often contain high-quality data for a given use case, such as safety and toxicity use cases, but are typically inaccessible to the broader research community.
OvalEdge can help R&D teams access the right data fast
In all of the above scenarios, addressing data issues such as data quality, completeness, and bias is crucial for maximizing the potential of AI to accelerate drug discovery and development processes. OvalEdge is a comprehensive, end-to-end data governance platform with all the tools researchers need to fuel AI algorithms with accurate, high-quality data.
We are focused on making data AI-ready. And here’s how we do it.
Our data catalog enables you to crawl and categorize all of the metadata in your ecosystem before centralizing access to it through a user-friendly, accessible interface. Using our data quality improvement tools, you can set enforceable data quality rules and monitor the quality of your data over time.
Data lineage capabilities enable you to take a deep dive into your data, identifying exactly where it comes from, where it's been, and if it's the right fit for the task at hand.
Ultimately, OvalEdge enables you to deal with data issues at the source as soon as possible before they infiltrate the data, models, and systems that drive your AI technologies.
Smart-scoping
Using AI for drug discovery is a fledgling process, but it begins with data. That’s why it’s important to start small and focus on targeted data governance or smart-scoping.
Instead of starting with data-poor therapeutic areas, such as infectious diseases, one way to smart-scope would be to begin leveraging AI where data is rich. For example, the BCG/Wellcome report suggests you could start in oncology or immunology and target mature use cases, such as small molecule design and optimization or target identification.
Schedule a Demo
Fill the information below to set up a demo.