Table of Contents
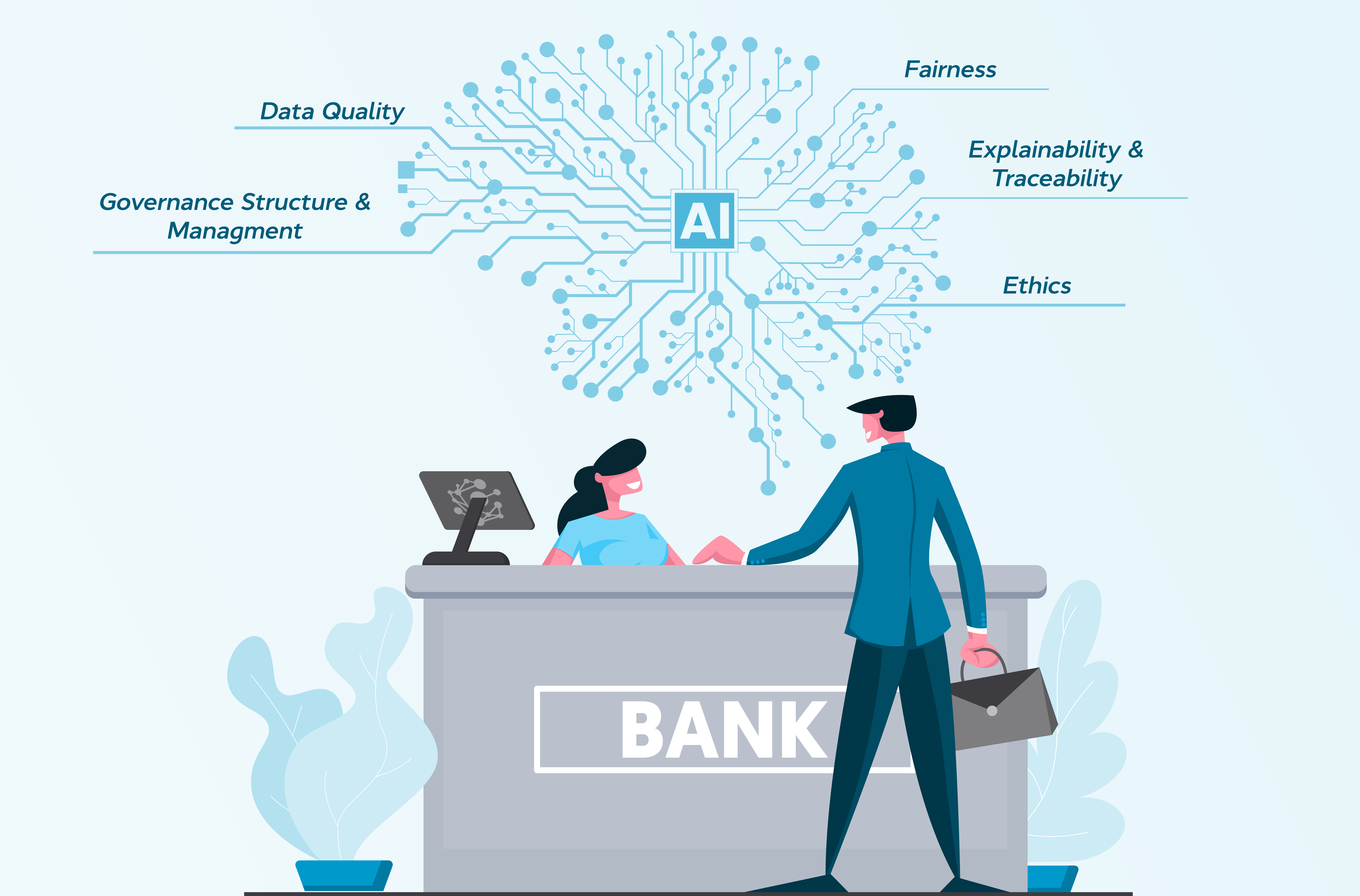
Importance of AI Governance in Banking
With AI adoption increasing dramatically in the banking industry, there is a growing need for comprehensive AI governance. In this article, we explore the most important governance activities for banks deploying AI technologies into their ecosystem.
AI Adoption is on the Rise
It seems like every day, another AI tool or solution is thrust onto the world stage. So, it should come as no surprise that AI adoption is accelerating, fast.
One industry that is being transformed by AI is banking. An upsurge in digital banking practices occurred during the Covid-19 pandemic. For example, Citibank reported a tenfold increase in Apple Pay activity during lockdown.
However, the pandemic was merely a catalyst, igniting a massive shift in customer expectations. This demand is being answered by AI technologies.
AI Governance is Critical in Banking
With AI set to shake the very foundations of the banking industry, AI governance is more important for the banking sector than almost any other. Although the essence of the risks involved in AI implementation for banks are no different from those in other industries, it is the outcomes that could materialize owing to these risk factors can be far more damaging.
Consumers, financial institutions, and even the global financial system can be severely affected. The most significant risks are related to bias. Ultimately, when data fed into AI banking systems is biased, decisions made could negatively affect specific customer groups.
Related Post: Trusted AI: Why AI Governance is a Business-Critical Concern
AI Governance in Banking
The best way to understand the core areas of AI governance in banking is to look at the value-chain activity. This activity can be broken down into six components: inputs, outputs, models, systems, processes, and policies.
Components of a Value-chain Activity in Banking
Inputs
- Data quality: The main challenge with bias avoidance is ensuring that decisions are made fairly. This can be achieved through existing data governance practices already deployed by a bank and should be applied to the data used in model training, testing, and validation.
Outputs
- Fairness: No decisions made using AI should negatively impact a particular user group. The core objective is to avoid discriminatory practices, such as denying a loan based on skewed data. To combat this, AI outputs and data sources must be reviewed and tested periodically.
Models
- Explainability and traceability: Any AI-driven decision that impacts the data provider must be explainable and all steps leading to that decision tracked. This must include thorough documentation and a ranking system that denotes the importance of each step in the decision-making process.
- Human in the loop: There must always be human oversight when AI models are deployed. This requires domain experts to regularly review AI systems and a series of boundaries put in place that determine the point at which human intervention is needed.
- Model security: Nefarious actors target AI models in unique ways, such as stealing the source code and introducing corrupted data. Banks must have robust cybersecurity systems in place to negate these threats.
Policy
- Ethics: AI in banking should be developed with ethical practices at the core of the design. To ensure these ethics are upheld, banks must establish an ethics committee to validate specific use cases, review ethics policies, and monitor outputs.
- Compliance: Model training requires a huge amount of customer data. There must be compliance policies in place that ensure the use of this data is compliant with data privacy, and other regulations, like the EU's GDPR. Ultimately, AI technologies must be compliant by design.
Related post: Why AI Governance Should Begin During Design, Not Deployment
Processes
- Governance structure and management: AI governance must mirror the other models of governance practices in a bank in their level of detail and enforcement. In fact, it should be integrated into the organization's business-wide risk management frameworks.
There must be well-defined roles and responsibilities for AI adoption that are assigned to specific team members and committees to ensure the overall success of AI governance. - Accountability: Human operators must have overall responsibility for AI decisions. This responsibility must lie with the banks’ boards of directors. AI standards and requirements must be consistently applied to AI systems developed in-house and acquired from third parties, and the accountability of the board must extend to all of these AI technologies.
- Skills: One of the key issues facing banks is a lack of individuals with the skills to build, maintain, oversee, and audit AI technologies. In particular, these skills must be held by senior management, risk management, and compliance teams. While it is tangible to source this expertise from outside sources, internal teams must have the required training too.
How Can OvalEdge Help?
AI data readiness in banking is a core focus at OvalEdge.
Which is why we have developed the dedicated governance tools required to accelerate secure AI governance in the banking sector.
Our data governance toolkit tackles input data issues at the source, and fast, ensuring data quality across your AI ecosystem, and helping you to avoid bias.
In terms of output, our governance tools enable you to govern your AI data effectively, trace its lineage, and more, so you can rest assured that your AI models are making fair decisions. We help you enforce compliance and other policies and establish roles and responsibilities while providing a solid, centralized foundation for all of your governance and management activities.
Schedule a Demo
Fill the information below to set up a demo.