Table of Contents
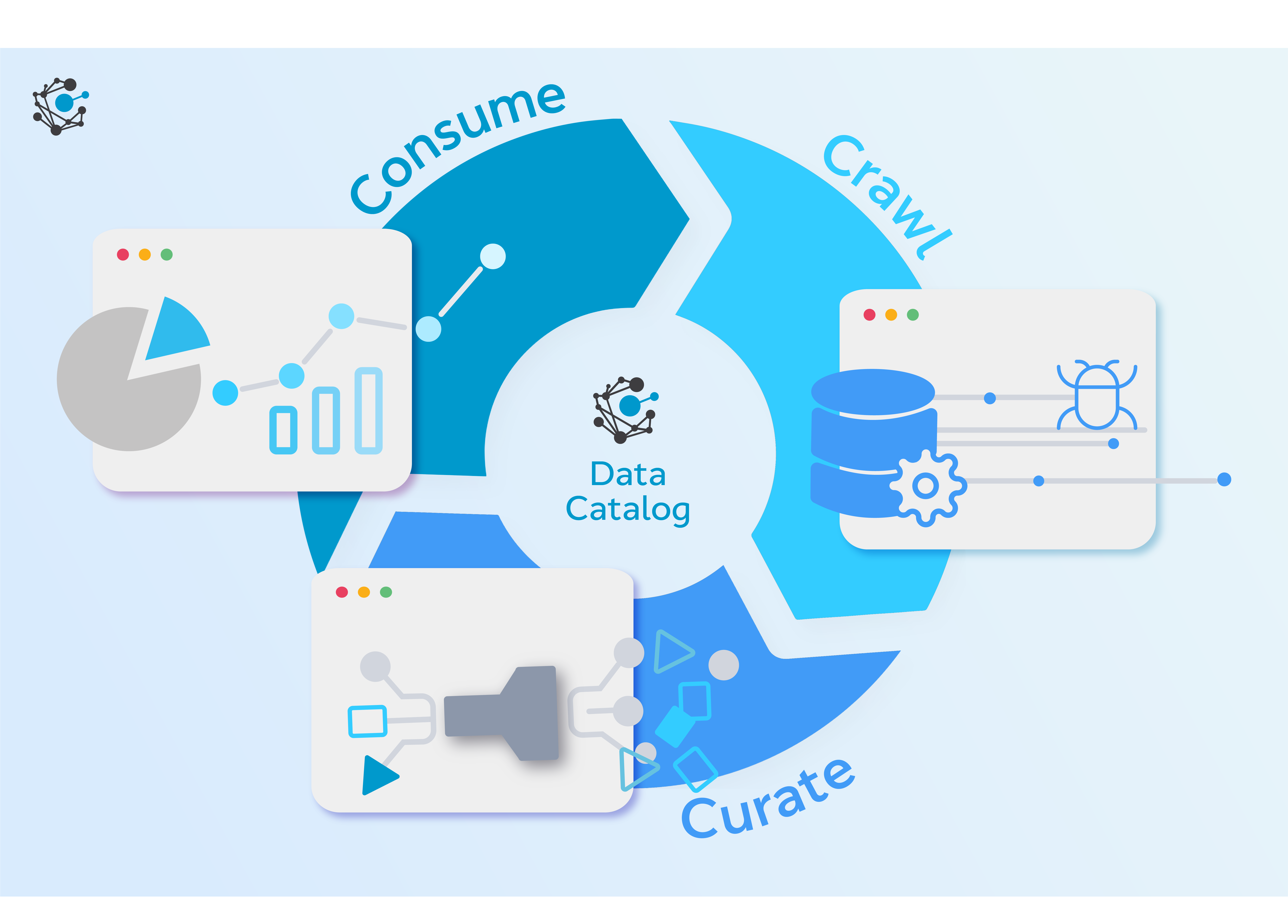
The Top Features of a Comprehensive Data Catalog
In the ever-evolving landscape of data management, organizations are increasingly recognizing the importance of having a robust data catalog to streamline the organization, discovery, and utilization of their data assets. A data catalog serves as a centralized repository that empowers teams to manage, curate, and consume data efficiently. Let's delve into the top features that make a data catalog indispensable in today's data-driven world.
Related Post: A step-by-step guide to build a data catalog
Data Catalog features can be broadly divided into three categories:
- Crawl,
- Curate &
- Consume.
1. Crawling Features
Crawl features ensure the automatic ingestion of metadata, data stats, etc., into a data catalog. The following features ensure stress-free ingestion into the data catalog.
Connectivity to Existing Data Ecosystem
A data catalog's crawl feature is the bedrock of its functionality. It involves establishing seamless connectivity to an organization's existing data ecosystem. This ensures the catalog can effectively index and manage data across diverse sources, including databases, data warehouses, and cloud storage.
Ability to connect to various kinds of data sources:
- Databases like Oracle, MySQL, SQL Server, etc.
- Data Lakes like S3, Hadoop.
- Data Warehouses like Snowflake, Databricks, BigQuery, Redshift.
- Reporting Systems like Business Objects, etc.
- Self-service analytics like Qlik, Tableau, PowerBI, and SSAS.
- Streaming Platforms. like Kafka, etc.
- Source Applications like SAP and Salesforce.
- AI systems like AzureML, etc.
- ETL systems like SSIS, Talend, and Informatica.
Bringing Desired Metadata
Crawling extends beyond mere data collection; it involves extracting and bringing in the desired metadata. This includes essential information about the data, such as source, format, schema, and data types. The catalog's ability to comprehensively gather metadata contributes significantly to effective data organization.
Bringing Active Metadata
Active metadata refers to real-time information about data usage, performance, and changes. A data catalog's ability to capture and present active metadata provides users with up-to-date insights into the dynamics of their data assets.
Related Post: What is Active Metadata Management? Definition & Benefits
2. Curation Features
Curate means that users can extend metadata and acquire knowledge from individuals. While curation covers a wide range of functionality, here are some of the top features to take into account to introduce automation and algorithms into the curation process.
Automatic Curation via Algorithms
Efficient curation is essential for making data discoverable and usable. Algorithms play a crucial role in automating the curation process. They assist in organizing and categorizing data based on predefined rules and criteria.
Algorithms for PII Identification
Data catalogs incorporate personally identifiable information (PII) identification algorithms to address privacy concerns. This ensures sensitive data is appropriately handled and protected.
Algorithms for Lineage
Understanding the lineage between different data entities is vital. Comprehending the origins and destinations of data is crucial in a data catalog. This requirement is essential for compliance and plays a significant role in building trust with data consumers. There are various ways in which algorithms can be developed:
- Query logs: This approach is effective for connectors like Snowflake, but it may not work well for Tableau, PowerBI, etc.
- Parsing source code: This is one of the most trusted ways to build lineage. A data catalog must provide end-to-end data lineage, as any breakage in connectivity defeats the purpose.
- Audit logs: Some catalogs build lineage by examining audit logs. However, this technique is not preferable as it depends on whether the connector has audit log capabilities.
Algorithms for Entity Relationships Diagram
Understanding the lineage and relationships between different data entities is vital. Algorithms that create visual representations, such as entity relationship diagrams, offer valuable insights into data dependencies and connections.
AI Algorithms for Automatic Writing
Incorporating artificial intelligence (AI) algorithms for automatic writing simplifies the creation of descriptive metadata. This feature enhances the catalog's ability to provide detailed information about each dataset.
Related Post: 4 Steps to AI-Ready Data
Algorithms for Duplicate Detections
Duplicate data can lead to confusion and errors. Algorithms for duplicate detection automatically identify and handle redundancies, maintaining data integrity.
Algorithms for Data Quality Score, Popularity, and Importance
Algorithms assessing data quality, popularity, and importance contribute to effective prioritization and decision-making. They enable users to focus on high-quality, relevant data for their tasks.
Assignment of Work to Various Responsible People
A data catalog facilitates the assignment of responsibilities, ensuring that individuals or teams are accountable for specific data assets. This feature streamlines collaboration and ensures efficient data management.
3. Consume Features
Consume features are designed for end-users to easily access and utilize the data. A data catalog doesn’t help the organization unless users can navigate and gain insights from the solution. Remember these next features so you don’t forget the user’s experience.
Algorithms for Easy Data Discovery
Intuitive algorithms for data discovery simplify the process of finding relevant datasets. Users can explore the catalog effortlessly, uncovering the information they need.
Data Search and Filtering Capabilities
Robust search and filtering capabilities enhance user experience, allowing for quick and precise retrieval of data. Users can tailor their searches based on various criteria.
Navigational Capabilities Using Data Grid
Data grids provide a visual representation of datasets, enhancing navigational capabilities. Users can easily explore relationships between different datasets within the catalog.
Integration with Existing Data Ecosystem and API Availability
Seamless integration with the existing data ecosystem and the availability of APIs enable interoperability. Users can leverage the catalog's features within their existing workflows and applications.
Data Access Cart for Buying Data
For organizations with data monetization strategies, a data access cart feature facilitates the buying and selling of data assets, promoting a transparent and controlled data exchange.
4. Security & Administration
Safeguarding data assets is as important as crawling, curating, and consuming data. Here are some considerations when examining a data catalog’s security and administration areas.
Roles-Based Security and User License-Based Security
Data security is paramount. Roles-based and user license-based security ensure that only authorized personnel have access to specific data, safeguarding against unauthorized use.
Consideration of Factors: Size, Need, Budget, Integration
An effective data catalog takes into account various factors, such as the organization's size, specific needs, budget constraints, and seamless integration with the existing data ecosystem. This ensures a tailored and efficient solution.
In conclusion, a comprehensive data catalog is a cornerstone for organizations aiming to harness the full potential of their data. By seamlessly navigating, curating, and consuming data, these catalogs empower teams to make informed decisions, drive innovation, and stay ahead in today's data-driven landscape.
Book a call with us to find out:
|
Schedule a Demo
Fill the information below to set up a demo.